For the pdf slides, click here
K-means Clustering vs Mixtures of Gaussians
K-means clustering
K-means clustering: problem
Data
- \(D\)-dimensional observations: \(\mathbf{x}_1, \ldots, \mathbf{x}_N\)
Parameters
- \(K\) clusters’ means: \(\boldsymbol\mu_1, \ldots, \boldsymbol\mu_K\)
- Binary indicator \(r_{nk} \in \{0, 1\}\): if object \(n\) is in class \(k\)
Goal: find values for \(\{\boldsymbol\mu_k\}\) and \(\{r_{nk}\}\) to minimize the objective function (called a distortion measure) \[ J = \sum_{n=1}^N \sum_{k = 1}^K r_{nk} \|\mathbf{x}_n - \boldsymbol\mu_k \|^2 \]
K-means clustering: solution
Two-stage optimization
- Update \(r_{nk}\) and \(\boldsymbol\mu_k\) alternatively, and repeat until convergence
- Resembles the E step and M step in the EM algorithm
E(expectation) step: updates \(r_{nk}\).
- Assign the \(n\)th data point to the closest cluster center \[ r_{nk} = \begin{cases} 1 & \text{ if } k = \arg\min_j \|\mathbf{x}_n - \boldsymbol\mu_k \|^2\\ 0 & \text{ otherwise} \end{cases} \]
M(maximization) step: updates \(\boldsymbol\mu_k\)
- Set cluster mean to be mean of all data points assigned to this cluster \[ \boldsymbol\mu_k = \frac{\sum_{n} r_{nk} \mathbf{x}_n}{\sum_{n} r_{nk}} \]
Mixture of Gaussians
Mixture of Gaussians: definition
Mixture of Gaussians: log likelihood \[\begin{equation}\label{eq:mixture_of_gaussians} \log p(\mathbf{x}) = \log \left\{ \sum_{k = 1}^K \pi_k \cdot \text{N}\left(\mathbf{x} \mid \boldsymbol\mu_k, \boldsymbol\Sigma_k \right) \right\} \end{equation}\]
Introduce a \(K\)-dim latent indicator variable \(\mathbf{z}\in \{0, 1\}^K\) \[ z_k = \mathbf{1}(\text{if $\mathbf{x}$ is from the $k$-th Gaussian component}) \]
The marginal distribution of \(\mathbf{z}\) is multinomial \[ p(z_k = 1) = \pi_k \]
- We call the posterior probability as the Responsibility that component \(k\) takes for explaining the observation \(\mathbf{x}\) \[ \gamma(z_k) = p(z_k = 1\mid \mathbf{x}) = \frac{\pi_k \cdot \text{N}\left(\mathbf{x} \mid \boldsymbol\mu_k, \boldsymbol\Sigma_k \right)} {\sum_{j=1}^K \pi_j \cdot \text{N}\left(\mathbf{x} \mid \boldsymbol\mu_j, \boldsymbol\Sigma_j \right)} \]
Mixture of Gaussians: singularity problem with MLE
Problem with maximum likelihood estimation: presence of singularities: there will be clusters that contains only one data point, so that the corresponding covariance matrix will be estimated at zero, thus the likelihood explodes
Therefore, when finding MLE, we should avoid finding such singularity solution and instead seek well-behaved local maxima of the likelihood function: see the following EM approach
Alternatively, we can to adopt a Bayesian approach
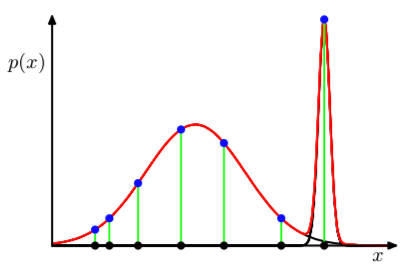
Figure 1: Illustration of singularities
Conditional MLE of \(\boldsymbol\mu_k\)
- Suppose we observe \(N\) data points \(\mathbf{X} = \{\mathbf{x}_1, \ldots, \mathbf{x}_N\}\)
Similarly, we write the \(N\) latent variables as \(\mathbf{Z} = \{\mathbf{z}_1, \ldots, \mathbf{z}_N\}\)
Set the derivatives of \(\log p(\mathbf{X} \mid \boldsymbol\pi, \boldsymbol\mu, \boldsymbol\Sigma)\) with respect to \(\boldsymbol\mu\) to zero \[ 0 = \sum_{n=1}^N \gamma(z_{nk}) ~\boldsymbol\Sigma_k ~\left(\mathbf{x}_n - \boldsymbol\mu_k \right) \] Then we obtain \[ \boldsymbol\mu_k = \frac{1}{N_k} \sum_{n=1}^N \gamma(z_{nk})~ \mathbf{x}_n \] where \(N_k\) is the effective number of points assigned to cluster \(k\) \[ N_k = \sum_{n=1}^N \gamma(z_{nk}) \]
Conditional MLE of \(\boldsymbol\Sigma_k\) and \(\pi_k\)
Similarly, setting the derivatives of log likelihood wrt \(\boldsymbol\Sigma_k\), we have \[ \boldsymbol\Sigma_k = \frac{1}{N_k} \sum_{n=1}^N \gamma(z_{nk})~ \left(\mathbf{x}_n - \boldsymbol\mu_k\right)\left(\mathbf{x}_n - \boldsymbol\mu_k\right)^\top \]
Use Lagrange multiplier to maximize log likelihood wrt \(\pi_k\) under the constraint that all \(\pi_k\) add up to one: \[ \log p(\mathbf{X} \mid \boldsymbol\pi, \boldsymbol\mu, \boldsymbol\Sigma) + \lambda \left( \sum_{k=1}^K \pi_k - 1 \right) \] we get the solution \[ \pi_k = \frac{N_k}{N} \]
The above results on \(\boldsymbol\mu_k, \boldsymbol\Sigma_k, \pi_k\) are not closed-form solution because the responsibilities \(\gamma(z_{nk})\) depend on them in a complex way.
EM algorithm for mixture of Gaussians
Initialize \(\boldsymbol\mu_k, \boldsymbol\Sigma_k, \pi_k\), usually using the \(K\)-means algorithm.
E step: compute responsibilities using the current parameters \[ \gamma(z_{nk}) = \frac{\pi_k \cdot \text{N}\left(\mathbf{x}_n \mid \boldsymbol\mu_k, \boldsymbol\Sigma_k \right)} {\sum_{j=1}^K \pi_j \cdot \text{N}\left(\mathbf{x}_n \mid \boldsymbol\mu_j, \boldsymbol\Sigma_j \right)} \]
M step: re-estimate the parameters using the current responsibilities, where \(N_k = \sum_{n=1}^N \gamma(z_{nk})\) \[\begin{align*} \boldsymbol\mu_k^{\text{new}} & = \frac{1}{N_k} \sum_{n=1}^N \gamma(z_{nk})~ \mathbf{x}_n\\ \boldsymbol\Sigma_k^{\text{new}} & = \frac{1}{N_k} \sum_{n=1}^N \gamma(z_{nk})~ \left(\mathbf{x}_n - \boldsymbol\mu_k\right)\left(\mathbf{x}_n - \boldsymbol\mu_k\right)^\top\\ \pi_k^{\text{new}} & = \frac{N_k}{N} \end{align*}\]
Check for convergence of either the parameters or the log likelihood. If not converged, return to step 2.
Connection between K-means and Gaussian mixture model
K-means algorithm itself is often used to initialize the parameters in a Gaussian mixture model before applying the EM algorithm
Mixture of Gaussians: soft assignment of data points to clusters, using posterior probabilities
\(K\)-means can be viewed as a special case of mixture of Gaussian, where covariances of mixture components are \(\epsilon \mathbf{I}\), where \(\epsilon\) is a parameter shared by all components.
- In the responsibility calculation, \[ \gamma(z_{nk}) = \frac{\pi_k \exp\{-\| \mathbf{x}_n - \boldsymbol\mu_k\|^2 / 2\epsilon \}} {\sum_j \pi_j \exp\{-\| \mathbf{x}_n - \boldsymbol\mu_j\|^2 / 2\epsilon \}} \] In the limit \(\epsilon\rightarrow 0\), for each observation \(n\), the responsibilities \(\{\gamma(z_{nk}), k = 1, \ldots, K\}\) has exactly one unity and all the rest are zero.
EM Algorithm
The general EM algorithm
EM algorithm: definition
Goal: maximize likelihood \(p(\mathbf{X} \mid \boldsymbol\theta)\) with respect to the parameter \(\boldsymbol\theta\), for models having latent variables \(\mathbf{Z}\).
- Notations
- \(\mathbf{X}\): observed data; also called incomplete data
- \(\boldsymbol\theta\): model parameters
- \(\mathbf{Z}\): latent variables, usually each observation has a latent variable
- \(\{\mathbf{X}, \mathbf{Z}\}\) is called complete data
- Log likelihood
\[
\log~p(\mathbf{X} \mid \boldsymbol\theta) =
\log\left\{ \sum_{\mathbf{Z}} p(\mathbf{X}, \mathbf{Z} \mid \boldsymbol\theta) \right\}
\]
- The sum over \(\mathbf{Z}\) can be replaced by an integral if \(\mathbf{Z}\) is continuous
- The presence of sum prevents the logarithm from acting directly on the joint distribution. This complicates MLE solutions, especially for exponential family.
General EM algorithm: two-stage iterative optimization
Choose the initial parameters \(\boldsymbol\theta^{\text{old}}\)
E step: since the conditional posterior \(p\left( \mathbf{Z} \mid \mathbf{X}, \boldsymbol\theta^{\text{old}} \right)\) contains all of our knowledge about the latent variable \(\mathbf{Z}\), we compute the expected complete-data log likelihood under it. \[\begin{align*} \mathcal{Q}(\boldsymbol\theta, \boldsymbol\theta^{\text{old}}) & = E_{\mathbf{Z} \mid \mathbf{X}, \boldsymbol\theta^{\text{old}}} \left\{\log p(\mathbf{X}, \mathbf{Z} \mid \boldsymbol\theta) \right\} \\ & = \sum_{\mathbf{Z}} p\left( \mathbf{Z} \mid \mathbf{X}, \boldsymbol\theta^{\text{old}} \right) \log p(\mathbf{X}, \mathbf{Z} \mid \boldsymbol\theta) \end{align*}\]
- M step: revise parameter estimate
\[
\boldsymbol\theta^{\text{new}} = \arg\max_{\boldsymbol\theta}
\mathcal{Q}(\boldsymbol\theta, \boldsymbol\theta^{\text{old}})
\]
- Note in the maximizing step, the logarithm acts driectly on the joint likelihood \(p(\mathbf{X}, \mathbf{Z} \mid \boldsymbol\theta)\), so the maximizating will be tractable.
Check for convergence of the log likelihood or the parameter values. If not converged, use \(\boldsymbol\theta^{\text{new}}\) to replace \(\boldsymbol\theta^{\text{old}}\), and return to step 2.
Gaussian mixtures revisited
- Recall that latent variables \(\mathbf{Z}\in \mathbb{R}^{N \times K}\) : \[ z_{nk} = \mathbf{1}(\text{if $\mathbf{x}_n$ is from the $k$-th Gaussian component}) \]
- Complete data log likelihood
\[
\log p(\mathbf{X}, \mathbf{Z} \mid \boldsymbol\mu, \boldsymbol\Sigma, \boldsymbol\pi)
= \sum_{n=1}^N \sum_{k=1}^K z_{nk}\left\{\log \pi_k +
\log \text{N}\left(\mathbf{x}_n \mid \boldsymbol\mu_k, \boldsymbol\Sigma_k \right) \right\}
\]
- Comparing this with incomplete data log likelihood in Eq , we have the sum over \(k\) and logarithm interchanged. Thus, the logarithm acts on Gaussian density directly.
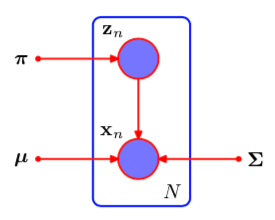
Figure 2: Mixture of Gaussians, treating latent variables as observed
Continue: Gaussian mixtures revisited
Conditional posterior of \(\mathbf{Z}\) \[ p(\mathbf{Z} \mid \mathbf{X}, \boldsymbol\mu, \boldsymbol\Sigma, \boldsymbol\pi) \propto \prod_{n=1}^N \prod_{k=1}^K \left[\pi_k \text{N}\left(\mathbf{x}_n \mid \boldsymbol\mu_k, \boldsymbol\Sigma_k \right) \right]^{z_{nk}} \] Thus, the conditional posterior of \(\{\mathbf{z}_n\}\) are independent
Conditional expectations \[ E_{\mathbf{Z} \mid \mathbf{X}, \boldsymbol\mu^{\text{old}}, \boldsymbol\Sigma^{\text{old}}, \boldsymbol\pi^{\text{old}}}~z_{nk} = \gamma(z_{nk})^{\text{old}} \]
Thus the objective function in the M-step \[\begin{align*} & E_{\mathbf{Z} \mid \mathbf{X}, \boldsymbol\mu^{\text{old}}, \boldsymbol\Sigma^{\text{old}}, \boldsymbol\pi^{\text{old}}}~ \log p(\mathbf{X}, \mathbf{Z} \mid \boldsymbol\mu, \boldsymbol\Sigma, \boldsymbol\pi)\\ = & \sum_{n=1}^N \sum_{k=1}^K \gamma(z_{nk})^{\text{old}}\left\{\log \pi_k + \log \text{N}\left(\mathbf{x}_n \mid \boldsymbol\mu_k, \boldsymbol\Sigma_k \right) \right\} \end{align*}\]